Some approaches to sparse solutions of linear ill-posed problems
With Elena Resmerita (Alpen-Adria University of Klagenfurt)
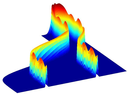
Some approaches to sparse solutions of linear ill-posed problems
During the past two decades it has become clear that $lp$ spaces with $p in (0,2)$ and corresponding (quasi)norms are appropriate settings for dealing with reconstruction of sparse solutions of ill-posed problems. In this context, the focus of our presentation is twofold. Firstly, since the question of how to choose the exponent $p$ in such settings has been not only a numerical issue, but also a philosophical one, we present a more flexible way of (performing/achieving) sparse regularization by varying exponents. Rather than using norms or quasinorms, we employ F-norms on infinite dimensional spaces. Secondly, we approach the ill-posed problem $Au=f$ by appropriate discretization in the image space. We formulate the so-called least error method in an $l1$ setting and perform the convergence analysis by choosing the discretization level according to both a priori and a posteriori rules.
Convergence rates are obtained under source condition (usually) yielding sparsity of the solution.
Joint research with Kristian Bredies, Barbara Kaltenbacher and Dirk Lorenz
- Speaker: Elena Resmerita (Alpen-Adria University of Klagenfurt)
- Thursday 12 July 2018, 15:00–16:00
- Venue: MR 14.
- Series: Applied and Computational Analysis; organiser: Carola-Bibiane Schoenlieb.