CCIMI Seminars
Relevance Forcing
With Christian Etmann (University of Bremen)
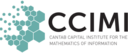
Relevance Forcing: More Interpretable Neural Networks through Prior Knowledge
Neural networks are able to reach high accuracies across many different classification tasks. However, these ‘black-box models’ suffer from one drawback: it is generally difficult to assess how the network reached its classification decision. Nevertheless, through different relevance measures, it is possible to determine which parts of the given input contribute to the resulting output. By imposing certain penalties on this relevance, through which we can encode prior information about the problem domain, we can train models which take this information into account. If we view these relevance measures as discretized dynamical systems, we may get some insight on the reliability of their explanation.
- Speaker: Christian Etmann (University of Bremen)
- Wednesday 09 May 2018, 15:30–16:30
- Venue: MR3 Centre for Mathematical Sciences.
- Series: CCIMI Seminars; organiser: Rachel Furner.