CCIMI Seminars
Randomised dimensionality reduction for persistent homology
With Martin Lotz – University of Manchester
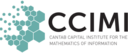
Randomised dimensionality reduction for persistent homology
We discuss ways in which ideas arising from compressive sensing and related fields can lead to complexity reductions in topological data analysis. In particular, it is possible to reduce the computation of persistent homology of Euclidean point clouds to spaces whose dimension is proportional to an intrinsic notion of dimension, the Gaussian width, associated to structural properties of the data. We give an overview of the relevant theory and discuss applications and limitations of this approach.
- Speaker: Martin Lotz – University of Manchester
- Wednesday 01 November 2017, 14:00–15:00
- Venue: MR5 Centre for Mathematical Sciences.
- Series: CCIMI Seminars; organiser: Rachel Furner.