Homotopy methods for convex optimization
With Andreas Klingler (Innsbruck)
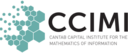
Homotopy methods for convex optimization
Convex optimization concerns the problem of finding the maximum of a linear function over a convex set. This class covers many optimization problems in quantum information, portfolio optimization, and machine learning.
In this talk, we will introduce a new approach to solving convex optimization problems via a homotopic approach. In this approach, we deform an optimization problem with a trivial solution into the target problem and keep track of the solutions along the homotopy. This is motivated by the field of numerical algebraic geometry, which solves systems of polynomial equations using a similar idea.
We show that our method applies to certain convex optimization problems, including Semidefinite Programs, Hyperbolic Programs, and convex optimization problems with a single convexity constraint. Moreover, we present several benchmark problems in which this method outperforms known methods.
- Speaker: Andreas Klingler (Innsbruck)
- Wednesday 07 February 2024, 14:30–15:30
- Venue: Centre for Mathematical Sciences MR11, CMS.
- Series: CCIMI Seminars; organiser: Hamza Fawzi.