Generative model-based super resolution and quality control for cardiac segmentation
With Shuo Wang (Digital Medicine Research Centre, Fudan University)
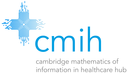
Generative model-based super resolution and quality control for cardiac segmentation
In cardiac imaging, a high-resolution geometric representation of the heart is desired for accurate assessment of its anatomical structure and function. This is not easily available due to the limit of acquisition duration and respiratory/cardiac motion in clinical practice. Stacks of multi-slice 2D images are usually acquired in clinical routine and segmentation of these images provides a low-resolution representation of cardiac anatomy, which may contain artefacts caused by motion. Here we propose a novel latent optimisation framework that jointly performs motion correction and super resolution for cardiac image segmentations based on generative learning. Moreover, quality control of the automatic segmentation results is realised via the proposed framework.
Zoom link:
https://maths-cam-ac-uk.zoom.us/j/92575403744?pwd=RHhqWC9wcUVWQi9xSzc1UE9BVGk3Zz09
Meeting ID: 925 7540 3744
Passcode: 974971
- Speaker: Shuo Wang (Digital Medicine Research Centre, Fudan University)
- Tuesday 29 June 2021, 13:00–14:00
- Venue: Virtual (see abstract for Zoom link).
- Series: CMIH Hub seminar series; organiser: J.W.Stevens.