Domain-theoretic Semantics for Dynamical Systems
With Levin Hornischer
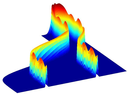
Domain-theoretic Semantics for Dynamical Systems: From Analog Computers to Neural Networks
Despite great empirical success, we are still lacking a theory of modern artificial intelligence. In particular, we are missing an interpretation of the ‘sub-symbolic’ computation performed by neural networks. For digital computation, this problem was solved by semantics: the mathematical description of the meaning of program code. In this paper, we work toward an analogous semantics for neural networks and other forms of ‘non-symbolic’ computation like analog computers—which all can be regarded as dynamical systems. To do so, we first summarize the three semantics for digital computation (operational, denotational, logical), and then develop their counterparts for non-symbolic computation (dynamical systems, domains, and modal logic). The key idea is to represent the dynamics of non-symbolic computation as a limit of finite symbolic approximations, which are given by interpretable observations. In an implementation, we thus illustrate the training dynamics of a neural network in a standard machine learning task.
- Speaker: Levin Hornischer
- Thursday 07 November 2024, 15:00–16:00
- Venue: Centre for Mathematical Sciences, MR14.
- Series: Applied and Computational Analysis; organiser: Matthew Colbrook.