Deep Learning Enables Prostate MRI Segmentation and Cancer Classification
With Yongkai Liu, David Geffen School of Medicine, UCLA
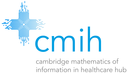
Deep Learning Enables Prostate MRI Segmentation and Cancer Classification
Prostate cancer (PCa) is the most common solid noncutaneous cancer in American men. Multiparametric MRI (mpMRI), including T2, diffusion weighted imaging (DWI) and T1 dynamic contrast enhanced imaging (DCE) has shown promising results for the detection and staging for clinically significant PCa (csPCa). The Prostate Imaging Reporting and Data System version 2.1 (PI-RADSv2.1), an expert guideline for performance and interpretation of mpMRI for PCa detection, T2 and DWI images are used for primary interpretation of lesions in the PZ and TZ respectively for assigning a PI-RADS score to lesions detected on mpMRI. A robust deep learning-based method for reproducible, automatic segmentation of prostate zones (ASPZ) may enable the consistent assignment of mpMRI lesion location since manual segmentation of prostate zones is a dependent time-consuming process, dependent on reader experience and expertise. Moreover, segmentation outcomes from ASPZ are typically deterministic; there is a lack of knowledge on the confidence of the model. Providing uncertainties of the model can improve the overall segmentation workflow since it easily allows refining uncertain cases by human experts. In addition, the evaluation of current state-of-art deep learning methods was limited by relatively small sample size, ranging from tens to hundreds of MRI scans. It is relatively expensive to create large, continuous samples with manual segmentation of WPG , which limits the ability to test the DL models in a clinical setting. We also evaluated a previously developed attentive deep learning-based automatic segmentation model using a large, continuous cohort of prostate 3T MRI scans (n=3360) to test its feasibility in a clinical setting. Finally, PI-RADS requires a high level of expertise and exhibits a significant degree of inter-reader and intra-reader variability, likely reflecting inherent ambiguities in the classification scheme. Image texture analysis provides the spatial arrangement of intensities in the image and can be used to quantitatively describe the tumor heterogeneity, which can be the primary feature of csPCa. An automated classification of PCa using texture analysis may overcome the current challenges associated with PI-RADS but commonly suffers from the laborious handcrafted feature design process to fully capture the underlying image texture. Alternatively, with the development of deep learning in medical imaging, convolutional neural networks (CNNs) with texture analysis may further improve the accuracy of PCa classification without handcrafted feature engineering.
Join Zoom Meeting
https://maths-cam-ac-uk.zoom.us/j/96932950870?pwd=b1o2c2UxckVITlRlazJzY0laRmVHZz09
Meeting ID: 969 3295 0870
Passcode: DRHjehPj
- Speaker: Yongkai Liu, David Geffen School of Medicine, UCLA
- Wednesday 17 November 2021, 13:00–14:00
- Venue: Virtual (see abstract for Zoom link).
- Series: CMIH Hub seminar series; organiser: J.W.Stevens.